Sponsored
Overcoming bias in data annotation
Overcome data annotation bias with strategies for fair, accurate AI—using clear guidelines, diverse annotators, and tech tools.
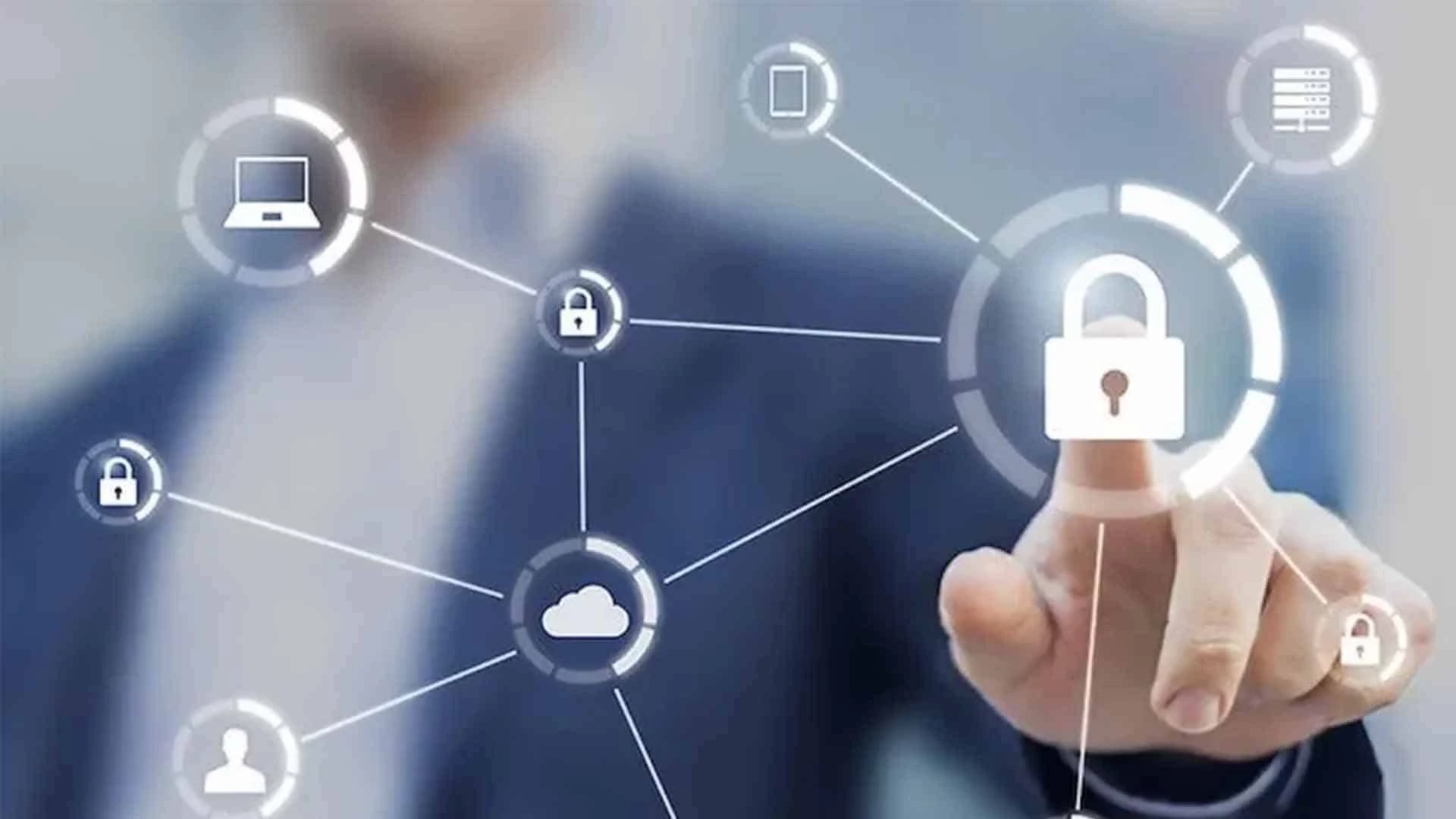
Just a heads up, if you buy something through our links, we may get a small share of the sale. It’s one of the ways we keep the lights on here. Click here for more.
In AI, annotated data directly shapes the accuracy and fairness of machine learning models. However, bias may lead to unreliable results and poor performance of machine learning models.
Let’s dive into the heart of this challenge and explore practical, actionable strategies to overcome bias in data annotation.
Understanding Bias in Data Annotation
First, what is data annotation, and how can bias affect it?
Data annotation is the process of labeling data, such as images, text, or videos, to train machine learning models.
Bias in this process happens when labels are influenced by personal opinions or outside influences. It’s a common trap, and if left unchecked, it can undermine the entire purpose of machine learning.
For instance, if a sentiment analysis model is trained with annotations that consistently rate phrases from a particular dialect as “negative,” it may perform poorly on texts written in that dialect.
Even worse, it may reinforce stereotypes, leading to ethical concerns and flawed AI results.
Types of bias in data annotation
Data annotation biases don’t manifest in just one way; they take different forms depending on the data source, annotators, and labeling instructions. Here are a few common culprits:
Sampling Bias
This happens when the collected data doesn’t include all the variety and diversity of the population. For example, training a facial recognition system on predominantly light-skinned faces can result in poorer accuracy for darker skin tones.
Annotator Bias
This arises from the annotators’ backgrounds, perspectives, or preconceptions. Two annotators viewing the same image or text can interpret it differently, especially if cultural or social differences are involved.
Labeling Bias
Often rooted in vague or subjective labeling instructions, this bias happens when the guidelines push annotators toward a particular interpretation.
Labeling images of athletes as “men” despite female representation, due to stereotype-driven labeling guidelines, is a classic example.
Strategies to minimize bias in annotation
Thankfully, bias doesn’t have to be inevitable. There are concrete steps you can take to keep bias at bay, ensuring your data annotation stays as neutral and representative as possible.
Develop Clear and Neutral Guidelines
Begin by crafting data annotation tech guidelines that leave minimal room for subjective interpretation.
Imagine annotating movie reviews if “negative” and “positive” are the only categories, but no specific guidance is provided, annotators may classify neutral or sarcastic reviews incorrectly.
Instead, offer explicit descriptions and examples for each label, even including edge cases.
Assemble a Diverse Annotator Pool
Think of your annotators as the lens through which your model will view the world. A narrow lens leads to narrow insights, so a homogenous annotator pool is likely to inject narrow perspectives.
By incorporating a diverse group of annotators from varied backgrounds, you’re more likely to capture a broad spectrum of views.
Provide Regular Training and Feedback
Training isn’t just for machines. Human annotators, too, benefit from periodic recalibration through training sessions and feedback loops.
Consistent, structured feedback, along with data annotation reviews from different perspectives, can help them recognize unintentional biases in their work.
Additionally, conducting bias-awareness workshops trains annotators to spot their own inclinations and make impartial decisions.
Just like a language that becomes more nuanced with practice, annotators’ labeling skills can grow sharper and more objective with proper guidance.
Use Cross-Annotation and Consensus Mechanisms
Let’s face it: no one’s perfect. Even the most diligent annotator can miss the mark sometimes. That’s where cross-annotation comes in handy.
By having multiple annotators work on the same data points, you can identify discrepancies and reach a consensus on ambiguous cases.
This not only balances individual biases but also gives you a more reliable label set.
This approach is especially useful for subjective categories, like emotion detection, where personal views often seep into annotation.
Leveraging Technology to Combat Bias
Human effort alone may not catch every instance of bias, especially in large-scale annotation projects. That’s where technology and the expertise of a data annotation company can help you spot and address biases that might slip past human oversight.
Automated Quality Control Tools
Automated quality control tools are like the quality inspectors of the annotation line. These tools review labeled data for inconsistencies, flagging patterns that may indicate bias.
AI-Driven Anomaly Detection
Anomaly detection techniques offer another layer of protection. These algorithms detect outliers in your annotated data, flagging data points that deviate significantly from the norm.
By reviewing flagged cases, you can catch unusual labeling patterns that may indicate bias, such as over-labeling certain sentiments in texts from particular demographics.
Bias Audit Frameworks
Several frameworks are designed specifically for auditing datasets for potential biases. Bias auditing tools scan data for overrepresented or underrepresented classes, helping you achieve a balanced distribution.
Think of it as a spotlight, shining on areas where your data may be uneven. Periodically running these audits keeps your dataset in check and aligns it with ethical AI goals.
Best practices recap
Overcoming bias in expert annotation is an ongoing process. Below are some best practices you can revisit to maintain a high standard of objectivity in your data:
- Clear Guidelines: Precise, well-defined instructions with examples help eliminate ambiguity.
- Diverse Annotators: Ensure your annotator team is as representative as possible to capture varied perspectives.
- Cross-Annotation: Employ multiple annotators on ambiguous cases to balance subjectivity.
- Feedback Loops: Continuous training and feedback refine annotators’ understanding and reduce bias over time.
- Technological Aids: Quality control tools, anomaly detection, and bias audit frameworks all work to keep data unbiased.
Strategy | Objective | Example Use Case |
Clear Guidelines | Minimize subjective interpretation | Detailed rules for sentiment labeling |
Diverse Annotators | Capture broad perspectives | Multicultural team for NLP projects |
Cross-Annotation | Balance individual biases | Consensus on ambiguous cases in emotion detection |
Feedback Loops | Reduce bias through continuous improvement | Workshops for recognizing implicit biases |
Technological Aids | Detect bias patterns in large datasets | Automated quality control and anomaly detection tools |
Final Thoughts
Bias in data annotation is a challenge, but it’s one that can be tackled with careful planning and the right tools. By building a strong framework of diverse perspectives, clear guidelines, and advanced technologies, you’re setting your machine learning model on a solid, objective foundation.
Every effort counts, and as you refine your approach to annotation, you’re not only enhancing model accuracy but also contributing to the broader goal of ethical, unbiased AI.
After all, the success of your AI system is only as reliable as the data it learns from—so make that data as fair and balanced as possible.
Have any thoughts on this? Drop us a line below in the comments, or carry the discussion to our Twitter or Facebook.
Editors’ Recommendations:
Disclosure: This is a sponsored post. However, our opinions, reviews, and other editorial content are not influenced by the sponsorship and remain objective.
Follow us on Flipboard, Google News, or Apple News
